Class-agnostic Reconstruction of Dynamic Objects from Videos
Zhongzheng Ren* Xiaoming Zhao* Alexander G. Schwing
(*equal contribution)
University of Illinois at Urbana–Champaign (UIUC)
Neural Information Processing Systems (NeurIPS), 2021
Paper | Bibtex | Poster
Abstract
We introduce REDO, a class-agnostic framework to REconstruct the Dynamic Objects from RGBD or calibrated videos. Compared to prior work, our problem setting is more realistic yet more challenging for three reasons: 1) due to occlusion or camera settings an object of interest may never be entirely visible, but we aim to reconstruct the complete shape; 2) we aim to handle different object dynamics including rigid motion, non-rigid motion, and articulation; 3) we aim to reconstruct different categories of objects with one unified framework. To address these challenges, we develop two novel modules. First, we introduce a canonical 4D implicit function which is pixel-aligned with aggregated temporal visual cues. Second, we develop a 4D transformation module which captures object dynamics to support temporal propagation and aggregation. We study the efficacy of REDO in extensive experiments on synthetic RGBD video datasets SAIL-VOS 3D and DeformingThings4D++, and on real-world video data 3DPW. We find REDO outperforms state-of-the-art dynamic reconstruction methods by a margin. In ablation studies we validate each developed component.
Method
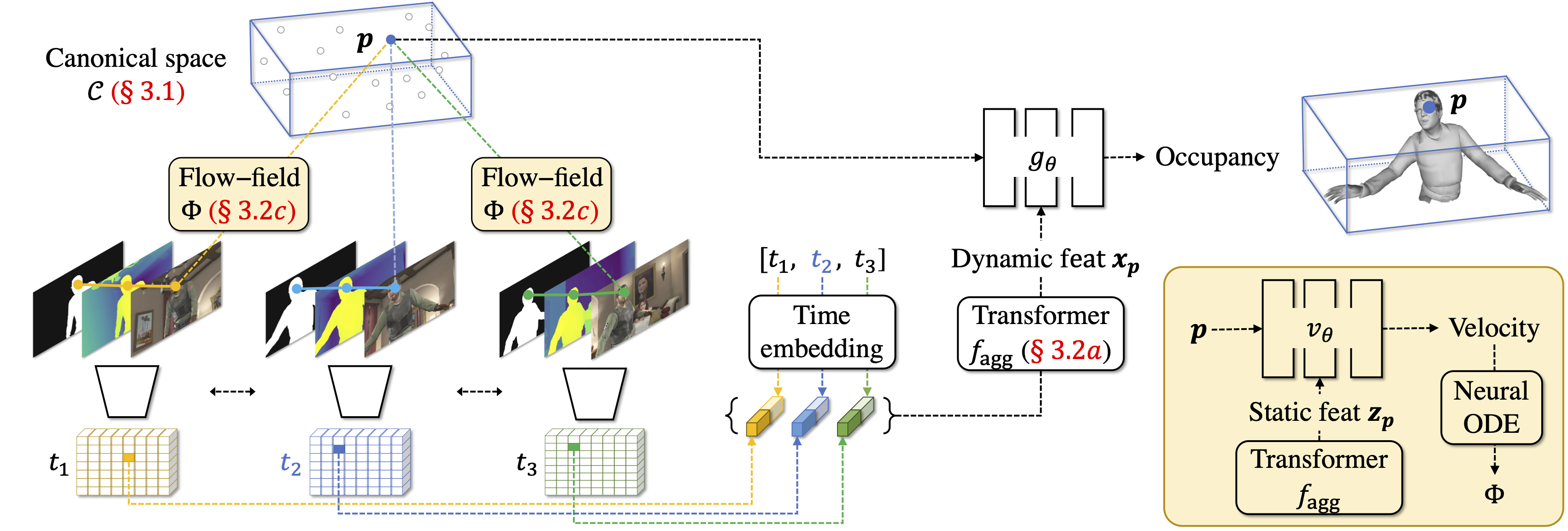
REDO Overview. For a query point p in canonical space, REDO first computes the pixel-aligned features from the feature maps of different frames using the flow-field. It then aggregates these features using the temporal aggregator. The obtained dynamic feature is eventually used to compute the occupancy score for shape reconstruction.
Results
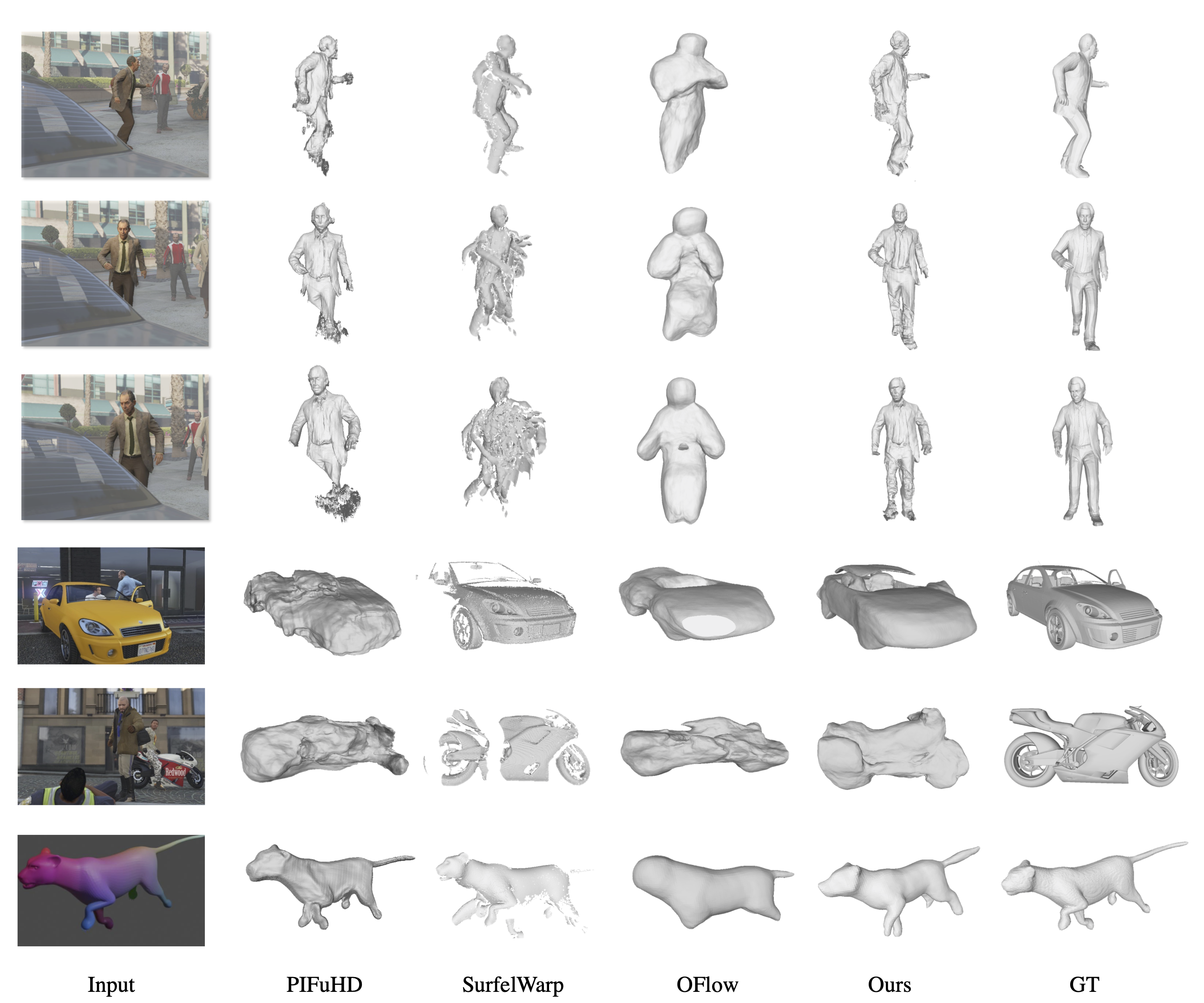
Visualization of REDO results. We illustrate the input frames, the reconstructed meshes obtained from different methods, and the ground-truth mesh.
Related Work
R. A. Newcombe, et al., DynamicFusion: Reconstruction and Tracking of Non-rigid Scenes in Real-Time. CVPR 2015.
Y. Hu, et al., SAIL-VOS 3D: A Synthetic Dataset and Baselines for Object Detection and 3D Mesh Reconstruction from Video Data. CVPR 2021.
M. Niemeyer, et al., Occupancy Flow: 4D Reconstruction by Learning Particle Dynamics. ICCV 2019.
S. Saito, et al., PIFuHD: Multi-Level Pixel-Aligned Implicit Function for High-Resolution 3D Human Digitization. CVPR 2020.
Acknowledgement
This work was supported in part by NSF under Grant #1718221, 2008387, 2045586, 2106825, MRI #1725729, NIFA award 2020-67021- 32799 and Cisco Systems Inc. (Gift Award CG 1377144 - thanks for access to Arcetri). ZR is supported by a Yee Memorial Fund Fellowship. We also thank the SPADE folks for the webpage template.